Effective adoption of AI tools in the lending industry is already showing clear benefits, but human oversight is still necessary
Business adoption of artificial intelligence (AI) is increasing rapidly, with a McKinsey report already putting the adoption rate at around 80%, with variations on degree of use and business sector. The importance of AI is undeniable, particularly in industries that have technology as a core competency, and Goldman Sachs estimates that Generative AI could increase global GDP by as much as 7%—around $7 trillion.
We have certainly seen this to be the case in the financial world, with lenders benefiting from AI adoption through the development of considerable competitive advantages, such as improved risk assessment, that will only become more pronounced and act as key differentiators within the market.
The term AI itself is a broad one, covering more than just the generative AI tools, such as ChatGPT, that have grown to prominence in the past few years. As far back as the 1980s we saw the introduction of expert systems—AI designed to emulate human decision-making, often through the use of “if-then rules”—and from around the turn of the millennium machine learning also became common place. Both of these tools continue to be widely used today.
There is, of course, the debate as to whether AI will be a net positive or negative for the world. For both parties, however, a consensus exists that, as AI technologies mature, their impact will be revolutionary, and we need to be ready to deal with that. Given the obvious importance of AI to businesses, and in this case specifically those in the credit industry, the key question is no longer whether to adopt, but how to properly harness the advantages it offers.
AI and lending
The credit market is a core facet of both domestic economies and the international economy as a whole, with bank and non-bank lenders—which include fintech lenders—playing a vital role in financing businesses and individuals alike. Lending decisions are made based on a wide range of factors, including income, credit history and character, and many of these decisions are now being made with AI assistance, and in some cases by AI alone.
The industry’s AI technology providers have focused on streamlining the lending process by helping banks make high-quality decisions efficiently, safely and sustainably. For example, Rich Data Corporation offers a Software as a Service (SaaS) platform that uses a blend of AI and human expertise to improve lenders’ decision making with transparency, compliance and accountability.
At the same time, we’re trying to improve equity of access to finances by improving the efficiency and accuracy in lending decisions. Given the importance of finance to our societal infrastructure, the ability for borrowers to access credit, and in turn resources, is integral to achieving whatever they set out to do, be that a business developing a product or an individual purchasing a home. From a lending perspective, aside from meeting the requirements of the equal opportunity legislation in place in many markets, providing equitable access can also offer an opportunity to grow the market and lower concentration risk and naturally help banks reduce their overall risk portfolio.
Benefits of AI
The impact of AI on lending processes and institutions so far has been quite clear. When assessing the more traditional measures of business success, such as revenue, costs and profit, McKinsey research clearly shows that adoption leaders are achieving higher returns in some or all categories.
Aside from these traditional metrics, there are also three other key benefits to adopting AI. Firstly, it can broaden the reach of a lender within or beyond its usual target markets. For example, for potential customers that don’t have a credit history and would have previously been off-limits, lenders can now use non-traditional data and AI techniques to make predictions on their creditworthiness. This is particularly useful in developing markets, where credit histories are not so common.
Secondly, AI data analysis can help with risk identification and increase accuracy of predictions, helping lenders reduce their overall risk exposure. US banks using AI, for example, will now be able to identify early warning signs of problematic market trends, for both small businesses as well as larger commercial firms and commercial real estate. Doing so will give banks a way to properly identify and manage credit risks, and potentially avoid significant credit losses.
The third benefit is relatively simple, and that is an increase in overall efficiency. Many AI techniques and tools can make the decision-making process significantly more streamlined, and in some cases automate it entirely, which helps lenders to reduce their costs.
There is also an increase in the recognition within the industry of the need for responsible lending, and this is something that AI can also help with. The lender often has the upper hand in the relationship, and exploitation of their borrowers can have an enormous impact. If lenders assess borrowers’ capability to repay accurately and efficiently with the assistance of AI and offer the most suitable loans, borrowers will get the most benefit from the loans and lenders will get higher lifetime value from these borrowers.
Implementation
There is still a clear difference in the current landscape between the leaders and laggards, but it is clear that even taking the first steps of technological adoption can have huge consequences on business outcomes. What is unclear, however, is the impact that generative AI may have when it becomes more widely used, in part due to its impact on knowledge work.
In the banking world, companies generally need data to be sourced and processed into a state where AI can be applied on top. The processes also require a lot of knowledge, usually coming from talent that knows different aspects of banking, how software systems generate and process the data and data discipline. There is also a need for a data-driven culture to help with adoption, because AI decisions are currently still driven by data.
Today, AI tools in lending streamline decision-making processes by combining calculative elements, like financial ratios, with behavioural factors like repayment and historical delinquency. Larger loans involve more human oversight and evaluations of character such as management capabilities, while simpler decisions can be automated. Continuous monitoring and audits are required to ensure the accuracy and fairness of AI decisions, identifying and addressing issues such as bias in facial recognition technology. AI’s integration with human expertise allows for flexible and controlled decision-making and implementation, allowing lenders to scale AI solutions as needed, maintaining control over core competencies and ensuring compliance with regulations.
The rapid evolution of AI also necessitates partnerships between lenders and AI vendors to stay competitive, which is why we see an increase in SaaS providers. There are a number of reasons for this: the total cost of ownership is lower; scaling-up usage is easier; switching vendors is relatively simple; and overall there is a flexibility and consistency to updates and technological developments without the need for extra in-house resources.
There is still some pushback against the SaaS model from some businesses, mostly due to the perception of where control lies in the partnership. SaaS vendors need to ensure that lenders still maintain control of the software and the underlying data, otherwise we will face internal concerns that things are moving away from them. For example, a previous client which was a major bank often discussed the idea of the “gravity of data”—where control of data lay—and the feeling that, because they saw data as their core competitive element, they needed to make sure it wasn’t falling outside of their business reach. We managed to reduce their concerns by setting up an instance in virtual private cloud with a major cloud provider and storing the data there.
In the past, the key competitor to SaaS solutions was on-premise software and in-house builds, but now, with AI advancing so quickly, such builds have sometimes become impossible to maintain and update to keep up with the new emerging technologies.
Difficulties and solutions
There are a number of difficulties associated with using AI in the lending industry and this differs between banks and non-bank lenders. Banks are regulated in a way that generally requires the models that they use to have high levels of transparency and explainability. There is a huge amount of compliance required to adopt a model, so dealing with the perceived lack of control or even a lack of understanding can be a major difficulty.
In our experience, building a model in the banking area normally takes weeks, but getting a model approved normally takes months and can even get up to 12 or 18 months if you need regulatory approval. It’s difficult, but obviously necessary, to manage risk and assuage cautions, given that banks are the heart of all financial systems. From the technology provider perspective, it is important to build in transparency from the start, rather than trying to tack it on at the end.
On the non-banking side, smaller companies have more trouble with AI adoption because they lack sufficient data to make the most accurate decisions. This is not a problem for larger companies such as PayPal or Alipay, but in general the majority of firms are smaller lenders. Unlike for banks, data privacy compliance can also be a major issue for non-bank lenders as there tends to be less oversight, meaning a greater temptation to misuse data for financial gain can exist.
Resisting that temptation and making everything controlled and compliant is generally quite difficult to balance. Here, we come back to the idea of responsible lending and responsible use of AI, and the difficulties of working out how to maximize lender profit as well as being fair to the borrower. Hopefully, we will see more governments implementing clear regulatory frameworks in this area to make this less of an issue.
There are also limitations to solely data-driven approaches, which were particularly evident during the COVID-19 pandemic, where historical data did not adequately represent future events due to a slew of unforeseen circumstances. This emphasized the importance of incorporating human expertise and reasoning to supplement data-drive models, allowing for more reliable decision-making. Considering scenarios and adjusting parameters to fit current challenges in this way will be particularly useful for tackling issues such as climate risk and supporting green lending, where historical data may be lacking.
Automation is possible and already in use for certain straightforward cases, but complex situations, such as freelancers with fluctuating incomes, may not be suitable for automated decisions. To prevent exploitation of the system, various controls and anti-fraud measures are in place, including verification processes and monitoring for unusual activities. The balance between automation and security is crucial, with lenders adjusting their level of automation based on the risk involved, such as lending of larger amount requiring stricter security measures.
Using technology to enhance lending processes ethically is crucial, and AI can be effectively utilized when objectives and constraints are clearly defined, but without well-defined processes, implementing AI in ethical lending can be challenging. AI as a tool should align with business principles and ethical considerations, with emphasis on data security, fairness and human-centered values.
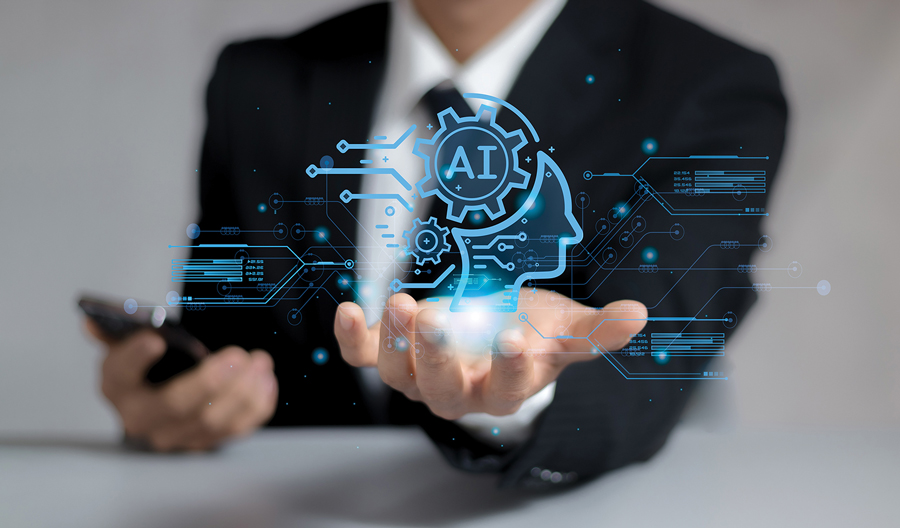
Growing role
AI’s role in lending will continue to grow, disrupting traditional processes and enhancing decision-making accuracy and efficiency overall. In the consumer sector, technological advancements in payments and transactions will continue to drive significant changes. On the lending side, AI, combined with human expertise, will challenge traditional roles, providing competitive advantages through improved decision-making processes.
AI is excellent for a number of reasons, but it has its limits, and whilst speeding up processes it raises issues about accuracy in the face of unexpected events and misuse of data by nefarious players. This means that AI’s integration into lending is not about replacing humans but about enhancing the capabilities and efficiency of financial institutions, and effective regulation is absolutely required. By balancing AI technology with human expertise, lenders should be in a position to ensure responsible and equitable lending practices, benefiting both lenders and borrowers in the long term.