How to extract the maximum value from your big data initiatives without falling into the hidden traps.
Everyone in the technology world can tell you how big data can help businesses do smarter marketing. Through the analysis of an enormous amount of data that was uncollectable before the internet age, marketers are better equipped than ever to grasp the pulse of consumers and target them with the most effective advertisements and other marketing materials.
However, applying real-life big data solutions is a much more complex and difficult process than you may think. For companies embracing the concept with great enthusiasm, the idea that big data is some sort of a shortcut to smart marketing is misleading. To reach the top level where business decisions are made on the basis of data analysis, companies need to navigate through a complicated system of data collection, data integration and management and the design of specific computing models.
Technology itself, which may revolutionize data gathering and computing, is not enough to make the entire system work. The key driver of a successful big data campaign is actually the people—their understanding of consumer behavior and experience of the industry are vital to the effectiveness of big data applications.
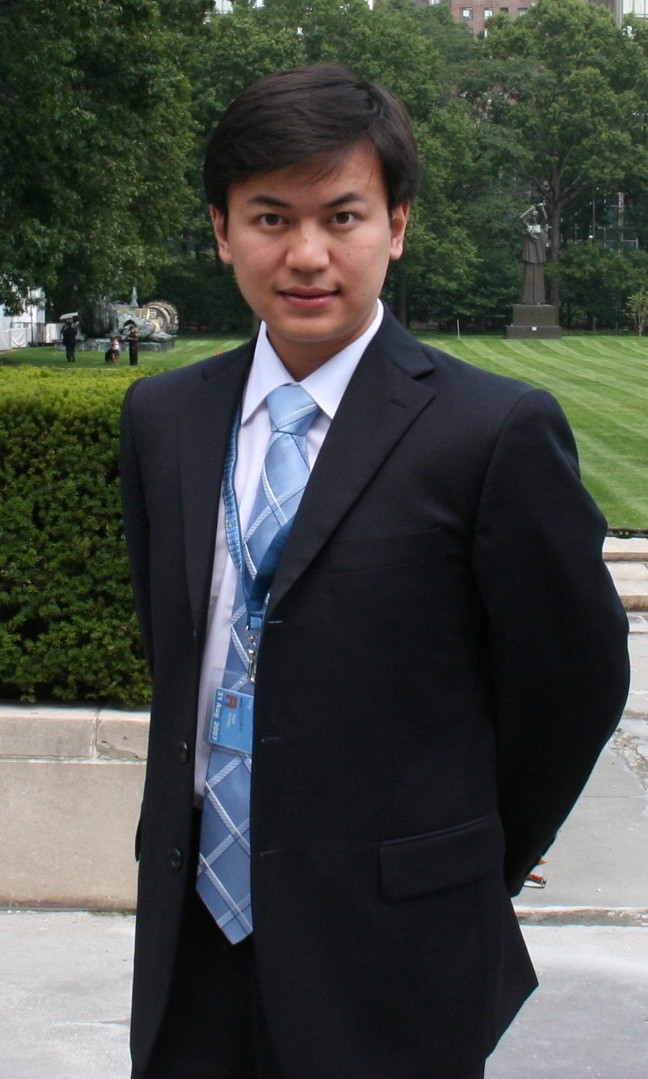
To illustrate why big data is not a panacea, we need to understand the following three traps that companies need to avoid when approaching big data solutions.
Noise
There’s a large variety of data available today that may be related to a business, but the key is to identify which data are more important to the decision making process.
For example in marketing, there are tons of factors that may influence consumers’ buying decisions, but it’s very hard to know how much each factor actually weighs. A couple of years ago, Nielson had a research project on how test-driving contributed to car sales in China and found that it would increase the chance of sales by as much as 40%. But they also found that the more expensive the cars were, the less important test-driving was for buyers. Therefore in this case, the test-driving data was probably just noise for luxury car brands.
On the other hand, however, the fact that there’s a lot of noise in your data doesn’t mean that you should just handpick certain kinds of data and overlook the others. For example, it’s probably not the most effective to display advertisements of a certain product to all people who searched for it on a shopping website; instead, you probably may want to see who actually read the comments under a specific product listing because it signals a higher interest to buy.
Process Sensitivity
There’s a long way to go between collecting data and getting a result from analysis—not necessarily in the sense of time, as computers are getting faster and faster, but in the sense of complexity. Algorithms are so complex nowadays and people may get very different results from the same set of parameters even if there’s only one difference between their mathematical models.
The simplest example is that to understand the central tendency of a data set, we can either calculate the mean or the median value. The mean value will be affected by the extreme values in the sample, while the median value will not. So when you design the computing model, you have to decide whether to keep the extreme values or to neglect them. Therefore depending on your hypothesis, you can get very different results from the same data sample.
There’s a saying that goes: “If you beat the data hard enough, you will get whatever results you want.” It may sound contradictory to the concept that big data is very objective—but in fact, it can very often be a very subjective process as well.
Causality
Many times we want to rely on big data to find the causality between events to help us make decisions. But how one event relates to another is not always that obvious and you may mistake what we call an exogenous cause with an endogenous cause.
For example, if we look at a group of people’s income data, we may draw the conclusion that the more educated a person is, the higher he or she is paid. What we do not see from the data is why some people have better education. One factor could be that they are faster learners, so they have higher academic achievements and also perform better at work. In this case, education is not the cause of higher income; it’s the learning ability that’s the endogenous cause of higher wages (of course we can look deeper to see what enhances people’s learning ability).
Here’s another example: if you look at the data of a search engine and find that Company A appearing at the top of certain search results receives the most clicks on the page, does that mean Company B should pay for that top spot and then expect to get a similar number of clicks? We can’t be sure because Company A may already have higher brand awareness and, therefore, it’s the brand that draws the most clicks on that page, not the top spot.
In summary, big data is a complex and systematic project that requires a fair amount of industry-specific expertise and experience. On the other hand, while big data may help us better understand consumer behavior, consumers themselves are evolving as well—they may actually hapmper companies’ data collection process, resulting in misleading data for marketers to work with. So companies need to keep in mind that the ultimate goal of using big data is less about peeking into the consumer’s brain, but more about providing them with greater value.
Read more about big data in China: