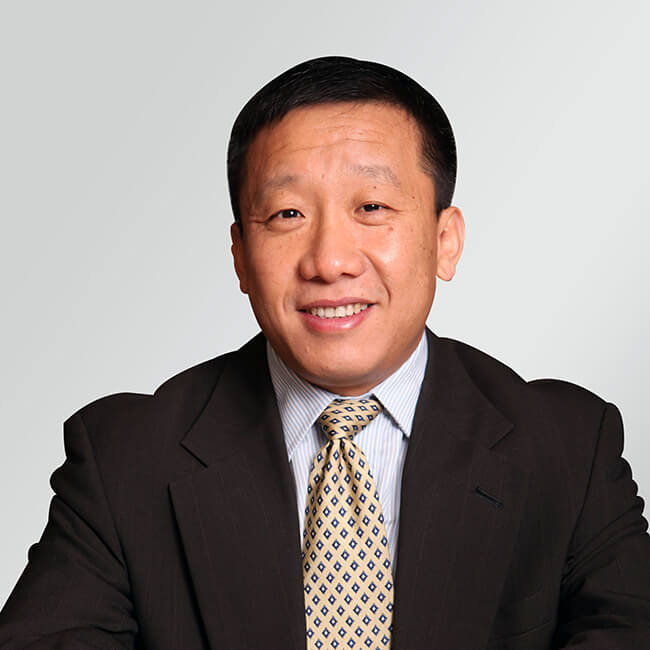
In the eyes of financial technology industry insiders, if you are not talking about “AI plus Finance,” then you are behind the times. Even worse, you risk being left behind, just as stubborn holdouts in another era were stranded when they failed to accept the internet.
AI (artificial intelligence) is not a new thing: it was first proposed as a field of research at a workshop at Dartmouth College in the United States in 1956. But the continuous evolution of algorithms, the maturation of hardware and the growing terabytes, petabytes and exabytes of data give AI hugely greater power today, especially as it relates to finance.
Traditionally, finance has two core functions: to lower transaction costs and to improve asset pricing. The widespread use of the internet has undermined the first by enabling more direct transactions, and AI is now disrupting the second by improving the speed and accuracy of asset pricing. Threatened by this are bank credit services, asset allocation by asset management firms, advisory services from investment and securities companies and the actuarial pricing services of insurance companies.
In the past, such financial institutions were protected by strong consumer “stickiness,” heavier capital investments and their first-mover advantage on data, and these factors still provide a bulwark against dramatic disruption. But significant change is coming and the threat to those who fail to adapt may come more from internet giants than from the emerging fintech (financial technology) players.
Changes Already Underway
Many financial institutions are building offerings around smart AI-based customer services. For example, Nordea Bank in Northern Europe has launched an AI-powered chatbot and virtual assistant called Nova that serves the bank’s network in Norway and other markets. Nova answers questions relating to the bank’s pensions and insurance business, and if it encounters a question it does not understand, it redirects the query to a human staff member.
In addition, some traditional financial institutions are now collecting customer information with AI technology. The authentication of customer identity information is an important part of risk control for financial institutions, and AI technology can enhance the efficiency of this process. For example, Ping An Insurance, the world’s largest insurance company by some measures, has developed live facial recognition technology that processes up to 30,000 faces per minute with an accuracy of 99.8%.
Such developments threaten the jobs of many employees at traditional banks. Nova and similar AI assistants are beginning to replace customer service staff throughout Europe and the United States, and job losses will increase as AI makes itself felt in other functions.
In the traditional loan approval process, a loan officer must collect the relevant information and then make a judgment call on whether to issue a loan. With AI technology, a far wider range of data is used for credit assessment. For example, traditional credit risk control models use only 20 to 30 variables, but data-based credit risk control models integrate more than 10,000 variables. Moreover, algorithmic methods such as “deep learning” can automatically identify specific variables relevant to credit performance that may be overlooked by traditional assessment frameworks. And AI is also playing an increasing role in fraud detection.
Yet, while AI technology is helping traditional financial institutions reduce staff numbers, save costs and improve efficiency, these gains only represent a small part of the subversive potential of AI in finance. The truly revolutionary aspect of the technology lies in how it will impact the two core functions of the financial industry: reducing transaction costs and asset pricing.
Real Subversion
The impact of AI on the second of the two functions will be significant: The entire financial industry should be on guard against the subversion of asset pricing. The pressure AI is exerting on the financial sector in this regard is greater than that of the internet on transaction costs.
The reason is that, for most financial institutions, effective asset pricing is more important to the business than reductions in transaction costs. For commercial banks, lending is the main source of profit, not the handling of payments. For insurance companies, the core business is the design and pricing of insurance products, which means that actuaries are the heart of the industry, not sales agents. For asset managers as well, success is primarily determined by the performance of investments and asset allocation. A good sales team is usually just a bonus.
Other aspects of the finance industry that have always been dependent on people are also threatened by AI, such as the investment advisory services offered by securities companies. Analysts are traditionally the soul of an investment advisory business. Based on their study of macroeconomic data and the financial data of listed companies, they produce research reports to provide customers with buy and sell stock recommendations. In the past, the analyst’s experience and judgment were important in determining their success and reputation, but AI is challenging this.
Algorithmic models working at high speed now produce fast assessments from the very latest economic variables and financial indicators. It is also possible to quickly find the point in history most similar to the current situation, and then produce a forecast based on past asset performance. Some platforms in the US, such as Automated Insights and Captricity, use AI to automatically generate analytics reports based on announcements from public companies, press coverage and even the latest news on social media (for example, President Trump’s tweets).
One of Wall Street’s best-known pioneers is Kensho, a data analytics company that deploys scalable machine learning and analytics systems to solve complex problems. For instance, Kensho can tell you, using historical data on the movements of the S&P and Dow Jones during Federal Reserve rate hikes, which industries will perform better or worse on the stock market when hikes occur. In the past, such predictions required long hours of hard labor by expensive analysts.
From the point of view of complexity and degree of specialization, the next target for AI subversion may be insurance. The mainstays of this industry, the actuaries who design and price insurance products, used to price insurance products mainly by dealing with probabilities such as survival, mortality, morbidity and so on.
Due to the complexity of the calculations, the actuarial business has not yet felt much of a technology shock, but the situation is changing because a core strength of AI lies in its ability to predict probabilities. With the massive amounts of data now available, it is likely that AI will soon change the business model of the actuarial industry by enabling more dynamic and accurate predictions of mortality and morbidity.
Don’t Panic
Although traditional financial institutions are feeling the impact of AI, there are three reasons for them not to panic.
First, consumer habits in the financial sector are “stickier” than in most other industries. After all, when it comes to big financial decisions, people are still more likely to trust someone they can talk to in person. For example, although the smart investment consulting industry can absorb many long-tail customers with small amounts of money, high-end private bank clients still demand the personal service of an account manager.
Second, AI requires heavy capital investment, and the strong capital position of financial institutions makes it possible for them to spend generously on IT. In the United States, JPMorgan Chase’s annual IT budget is $9.5 billion, Bank of America’s is $9 billion and Wells Fargo’s is $7 billion. Ping An’s annual R&D investment is more than RMB 7 billion ($1.1 billion). Emerging financial technology players cannot match this. At the end of the third quarter of 2016, the total invested by the US in AI startups was only $3.1 billion.
Third, the financial industry’s advantage as a first mover in data is even more obvious. Data is the foundation of AI and deep learning, and the need for confidentiality with financial data is high, and so the financial industry’s advantage is clear over any new challengers.
If the financial world’s big beasts make the most of these advantages, it could be the case that the brave new world of AI finance looks similar to the old one.